Using Limma to find differentially expressed genes
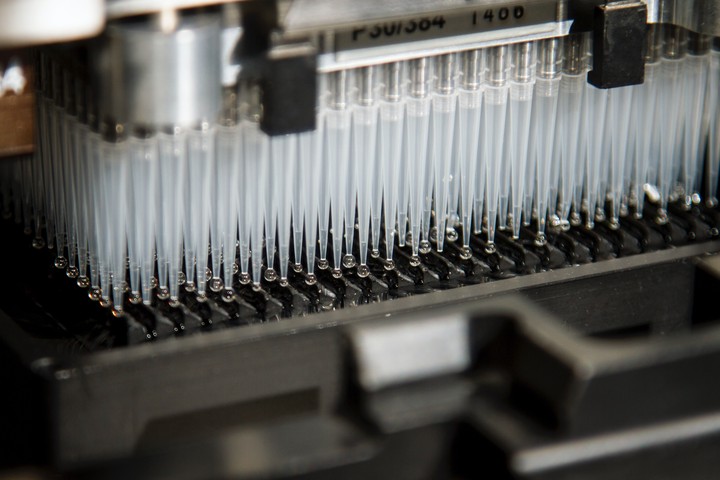
Ritchie, ME, Phipson, B, Wu, D, Hu, Y, Law, CW, Shi, W, and Smyth, GK (2015). limma powers differential expression analyses for RNA-sequencing and microarray studies.Nucleic Acids Research 43(7), e47.
limma is an R package hosted on Bioconductor which finds differentially expressed genes for RNA-seq or microarray. Recently I’ve been working on a PCR-based low-density array and noticed that I forgot how to use limma for the one hundredth time, so I decided to make a note.
Preparation
- Log-transformed expression data in a matrix:
Each column represents an experiement, and each row represents a detected gene/probe. - Design matrix:
Each column represents a status of your data (e.g., wild-type, mutant, rescued…), and each row corresponds to an experiment in the expression matrix. This could be generated from anexpressionDataset
object, or we could create this manually if you only have a raw expression dataset like me. - Contrast matrix: Specified a set of parameters to specify the contrasts being compared later.
library(limma)
# Create a dummy expression matrix
exp_matrix <- data.frame(sample_1 = c(3, 3, 2, 2, 1),
sample_2 = c(3.2, 2.8, 2, 2, 1.3),
sample_3 = c(1, 1, 2, 2.3, 5),
sample_4 = c(1.1, 0.9, 2, 2, 4),
sample_5 = c(2.5, 2.5, 2, 2.5, 2),
sample_6 = c(2.6, 2.3, 2.1, 2.2, 1.8),
row.names = paste("gene", seq(5), sep = "_"),
stringsAsFactors = FALSE)
print(exp_matrix)
## sample_1 sample_2 sample_3 sample_4 sample_5 sample_6
## gene_1 3 3.2 1.0 1.1 2.5 2.6
## gene_2 3 2.8 1.0 0.9 2.5 2.3
## gene_3 2 2.0 2.0 2.0 2.0 2.1
## gene_4 2 2.0 2.3 2.0 2.5 2.2
## gene_5 1 1.3 5.0 4.0 2.0 1.8
# Create a design matrix
design <- model.matrix(~ 0+factor(c(1,1,2,2,3,3)))
colnames(design) <- c("Wild_type", "Mutant", "Rescue")
print(design)
## Wild_type Mutant Rescue
## 1 1 0 0
## 2 1 0 0
## 3 0 1 0
## 4 0 1 0
## 5 0 0 1
## 6 0 0 1
## attr(,"assign")
## [1] 1 1 1
## attr(,"contrasts")
## attr(,"contrasts")$`factor(c(1, 1, 2, 2, 3, 3))`
## [1] "contr.treatment"
# Create a design matrix to indicate the comparisons to make by limma
cont_matrix <- makeContrasts(MutvsWT = Mutant-Wild_type,
MutvsRes = Mutant-Rescue,
levels=design)
print(cont_matrix)
## Contrasts
## Levels MutvsWT MutvsRes
## Wild_type -1 0
## Mutant 1 1
## Rescue 0 -1
Analysis
Now, I am pretty much ready (except for the need to understand the math).
# Fit the expression matrix to a linear model
fit <- lmFit(exp_matrix, design)
# Compute contrast
fit_contrast <- contrasts.fit(fit, cont_matrix)
# Bayes statistics of differential expression
# *There are several options to tweak!*
fit_contrast <- eBayes(fit_contrast)
# Generate a vocalno plot to visualize differential expression
# Highlighting the top 2 genes
volcanoplot(fit_contrast, highlight = 2)
# Generate a list of top 100 differentially expressed genes
top_genes <- topTable(fit_contrast, number = 100, adjust = "BH")
print(top_genes)
## MutvsWT MutvsRes AveExpr F P.Value adj.P.Val
## gene_1 -2.05 -1.50 2.233333 205.9703070 2.448629e-05 0.0001224315
## gene_2 -1.95 -1.45 2.083333 145.5778494 5.515948e-05 0.0001378987
## gene_5 3.35 2.60 2.516667 49.9796088 6.486868e-04 0.0010811446
## gene_4 0.15 -0.20 2.166667 2.6155372 1.715306e-01 0.2144132510
## gene_3 0.00 -0.05 2.016667 0.2942966 7.577324e-01 0.7577323813
# Summary of results (number of differentially expressed genes)
result <- decideTests(fit_contrast)
summary(result)
## MutvsWT MutvsRes
## Down 2 2
## NotSig 2 2
## Up 1 1